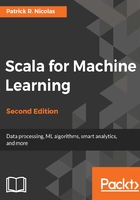
Moving averages
Moving averages provides data analysts and scientists with a basic predictive model. Despite its simplicity, the moving average method is widely used in a variety of fields such as marketing survey, consumer behavior, or sport statistics. Traders use the moving averages to identify levels of support and resistance for the price of a given security.
Note
Averaging reducing function:
Let's consider a time series xt = x(t) and a function f(xt-p-1,… xt) that reduces the last p observations into a value or average. The estimation of the observation at t is defined by the following formula:

Here, f is an average reducing function from the previous p data points.
Simple moving average
Simple moving average is the simplest form of the moving averaging algorithms [3:2]. The simple moving average of period p estimates the value at time t by computing the average value of the previous p observations using the following formula:
Note
Simple moving average:
M1: The simple moving average of a time series {xt} with a period p is computed as the average of the last p observations:

M2: The computation is implemented iteratively using the following formula:

Here, is the estimate or simple moving average value at time t.
Let's build a class hierarchy of moving average algorithms, with the parameterized trait MovingAverage
as its root:
trait MovingAverage[T]
We use the generic type Vector[T]
and the data transform with explicit configuration ETransform
introduced in the Explicit models section under Monadic data transformation in Chapter 2, Data Pipelines to implement the simple moving average, SimpleMovingAverage
:
class SimpleMovingAverage[[@specialized(Double) T: ToDouble]( period: Int)(implicit num: Numeric[T] //1 ) extends Etransform[Vector[T], DblVec](ConfigInt(period)) with MovingAverage[T] { val zeros = Vector.fill(0.0)(period-1) override def |> : PartialFunction[Vector[T], Try[DblVec]] = { case xt: Vector[T] if( xt.size >= period ) => { val (first, second) = xt.splitAt(period) //2 val slider = xt.take(xt.size - period).zip(second) //3 val c = implicitly[ToDouble[T]] val zero = first.sum/period //4 Try( zeros ++ slider.scanLeft(zero) { //5 case (s, (x,y)) => s + (c(y) – c(x))/period }) //6 } }
The class is parameterized for the type T
of elements of the input time series: we cannot make any assumption regarding the type of input data. The type of the elements of the output time series is Double
. The implicit instantiation of the class Numeric[T]
is required by the arithmetic operators sum
and /
(line 1). The implementation has a few interesting elements. First, the set of observations is split with the first period observations (line 2) and the index in the resulting clone instance is shifted by p observations before being zipped with the original to the array of pair of values: slider
(line 3):

Sliding algorithm to compute moving averages
The average value is initialized with the mean value of the first period
data points (line 4). The first period
values of the trends are initialized as zero (line 5). The method concatenates the initial null values and the computed average values to implement the formula M2 (line 5).
Weighted moving average
The weighted moving average method is an extension of the simple moving average by computing the weighted average of the last p observations [3:3]. The weights αj are assigned to each of the last p data point xj and normalized by the sum of the weights.
Note
Weighted moving average:
M3: Weighted moving average of a series {xt } with p normalized weights distribution {αj}:

Here, xt is the estimate or simple moving average value at time t.
The implementation of the WeightedMovingAverage
class requires the computation of the last p (weights.size)
data points. There is no simple iterative formula to compute the weighted moving average at time t+1 using the moving average at time t:
class WeightedMovingAverage[@specialized(Double) T: ToDouble]( weights: Features)(implicit num: Numeric[T] ) extends SimpleMovingAverage[T](weights.length) { //7 override def |> : PartialFunction[Vector[T], Try[DblVec]] = { case xt: Vector[T] if(xt.size >= weights.length ) => { val smoothed = (config to xt.size).map( i => xt.slice(i- config, i).zip(weights).map { // 8 case(x, w) => implicitly[ToDouble[T]].apply(x)*w}.sum //9 ) Try(zeros ++ smoothed) //10 } } }
The computation of the weighted moving average is a bit more involved than the simple moving average. Therefore, we specify the generation of byte code dedicated to Double
type using the @specialized
annotation. The weighted moving average inherits the class SimpleMovingAverage
(line 7) and therefore implements the explicit transformation ETransform
for a configuration of weights
, with input observations of type Vector[T]
and output of type DblVec
. The implementation of formula M3 generates a smoothed time series by slicing (line 8) the input time series and then computing the inner product of weights
and the slice of the time series (line 9).
As with the simple moving average, the output is the concatenation of the initial weights.size
null values, zeros
, and the smoothed
data (line 10).
Exponential moving average
The exponential moving average is widely used in financial analysis and marketing surveys because it favors the latest values. The older the value, the less impact it has on the moving average value at time t [3:4].
Note
Exponential moving average:
M4: The exponential moving average on a series {xt} and a smoothing factor α is computed by the following iterative formula:

Here, is the value of the exponential average at t.
The implementation of the ExpMovingAverage
class is rather simple. The constructor has a single argument, α
(decay rate) (line 11):
class ExpMovingAverage[@specialized(Double) T: ToDouble]( alpha: Double //11 ) extends ETransform[Vector[T], DblVec](ConfigDouble(alpha)) with MovingAverage[T]{ //12 override def |> : PartialFunction[Vector[T], Try[DblVec]] ={ case xt: Vector[T] if( xt.size > 0) => { val c = implicitly[ToDouble[T]] val alpha_1 = 1-alpha var y: Double = data(0) Try( xt.view.map(x => { val z = c.apply(x)*alpha + y*alpha_1; y = z; z }) ) //13 } } }
The exponential moving average implements the ETransform
with an input of type Vector[T]
and an output of type DblVec
( line 12). The method |>
applies the formula M4 to all observations of the time series within a map
(line 13).
The version of the constructor that uses the period p
to compute the alpha = 1/(p+1)
as an argument is implemented using the Scala apply
method:
def apply[T: ToDouble](p: Int): ExpMovingAverage[T] =
new ExpMovingAverage[T](2/(p + 1))
Let us compare the results generated from these three moving average methods with the original price. We use a data source, DataSource
, to load and extract values from the historical daily closing stock price of Bank of America (BAC) available at the Yahoo Financials pages. The class DataSink
is responsible for formatting and saving the results into a CSV file for further analysis. The DataSource
and DataSink
classes are described in detail in the Data extraction section under Source code considerations in the Appendix:
import YahooFinancials._ type DblSeries = Vector[Array[Double]] val hp = p >>1 val w = Array.tabulate(p)(n => if(n == hp) 1.0 else 1.0/(Math.abs(n - hp)+1)) //14 val sum = w.sum val weights = w.map { _ / sum } //15 val dataSrc = DataSource(s"$RESOURCE_PATH$symbol.csv",false)//16 val pfnSMvAve = SimpleMovingAverage[Double](p) |> //17 val pfnWMvAve = WeightedMovingAverage[Double](weights) |> val pfnEMvAve = ExpMovingAverage[Double](p) |> for { price <- dataSrc.get(adjClose) //18 if(pfnSMvSve.isDefinedAt(price) ) sMvOut <- pfnSMvAve(price) //19 if(pfnWMvSve.isDefinedAt(price) eMvOut <- pfnWMvAve(price) if(pfnEMvSve.isDefinedAt(price) wMvOut <- pfnEMvAve(price) } yield { val dataSink = DataSink[Double](s"$OUTPUT_PATH$p.csv") val results = List[DblSeries](price, sMvOut, eMvOut, wMvOut) dataSink |> results //20 }
Tip
isDefinedAt:
Each of the partial functions is validated by a call to isDefinedAt
. From now on, the validation of the partial function will be omitted throughout the book for the sake of clarity.
The coefficients for the weighted moving average are generated (line 14) and normalized (line 14). The trading data regarding the ticker symbol, BAC, is extracted from the Yahoo finances CSV file (line 16) YahooFinancials
using the adjClose
extractor (line 17). The next step is to initialize the partial functions pfnSMvAve
, pfnWMvAve
, and pfnEMvAve
related to each of the moving averages (line 18). The invocation of the partial functions with price
as argument generated the three smoothed time series (line 19).
Finally, a DataSink
instance formats and dumps the results into a file (line 20).
Tip
Implicit postfixOps:
The instantiation of the partial function filter |>
requires that the postfix operation postfixOps
be made visible by importing scala.language.postfixOps
.
The weighted moving average method relies on a symmetric distribution of normalized weights computed by a function passed as argument of the generic tabulate
method. Note that the original price time series is displayed if one of the specific moving averages cannot be computed. The following graph is an example of a symmetric filter for weighted moving averages:

Example of symmetric filter for weighted moving average
The three moving average techniques are applied to the price of the BAC over 200 trading days. Both the simple and weighted moving average use a period of 11 trading days. The exponential moving average method uses a scaling factor of 2/(11+1) = 0.1667:

11-day moving averages of Bank of America historical stock price
The three techniques filter the noise out of the original historical price time series. The exponential moving average reacts to sudden price fluctuation despite the fact that the smoothing factor is low. If you increase the period to 51 trading days (equivalent to 2 calendar months), the simple and weighted moving average produce a time series smoother than the exponential moving average with alpha = 2/(p+1) = 0.038:

51-day moving averages of Bank of America historical stock price
You are invited to experiment further with different smooth factors and weight distributions. You will be able to confirm the following basic rule: as the period of the moving average increases, noise with decreasing frequencies is eliminated.
In other words, the window of allowed frequencies is shrinking. The moving average acts as a low-pass filter that only preserves lower frequencies.
Fine-tuning the period of the smoothing factor is time-consuming. Spectral analysis, and more specifically the Fourier series, transforms a time series into a sequence of frequencies, which provides the statistician with a more powerful frequency analysis tool.
Tip
Moving average on multi-dimensional time series:
The moving average techniques are presented for single features or variable time series, for the sake of simplicity. Moving average on multi-dimensional time series are computed by executing a single variable moving average to each feature using the transform
method of Vector[T]
introduced in the first section. Let's take for example the simple moving average applied to a multi-dimensional time series xt
. The smoothed values are computed as follows:
val pfnMv = SimpleMovingAverage[Double](period) |> val smoothed = transform(xt, pfnMv)