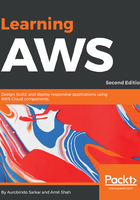
Understanding emerging cloud-based application architectures
In this section, we will describe common architecture patterns and deployment of some of the main processing models being used for batch processing, streaming applications, and machine learning pipelines. The underlying architecture for these processing models are required to support ingesting very large volumes of various types of data arriving at high velocities at one end, while making the output data available for use by analytical tools, reporting and modeling software, at the other.
The software platforms supporting such applications have the necessary features and support the key mechanisms required to access data across a diverse set of data sources and formats, and prepare it for downstream applications, either as low-latency streaming data or high-throughput historical data stores. For example, Apache Spark is an emerging platform that leverages distributed storage and processing frameworks to support querying, reporting, analytics and intelligent applications at scale.
The following figure shows a high-level architecture that incorporates these requirements in typical Spark-based batch and streaming applications:
