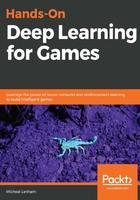
The present
At present, at least at the time of writing, we are still in the midst of a deep learning explosion with debris and chaos, and it is our job as developers to make sense of all this. Neural networks are currently the basis for many DL technologies, several of which we will cover in this book. Except, it seems that every day, new and more powerful techniques emerge, and researchers scramble to make sense of them. Now, this rush of ideas can actually stall a technology, as researchers spend more and more time trying to replicate results. It is most certainly a cause for much of the earlier stalling that ANNs (deep learning) previously suffered from. In fact, many skeptics in the industry are predicting yet another hiccup. So, should you be worried, is reading this book worth it? The short answer is yes. The long answer is probably not, this time things are very different and many deep learning concepts are now generating revenue, which is a good thing. The fact that DL technology is now a proven money-earner puts investors at ease and only encourages new investment and growth. Exactly how much growth is yet to be seen, but the machine and DL space is now ripe with opportunity and growth from all sectors.
So, is it still possible that the game industry will again turn its back on games? That is also unlikely, generally because many of the more recent major advances, like reinforcement learning, were built to play classic Atari games, and use games as the problem. This only encourages more research into deep learning using games. Unity 3D, the game platform, has made a major investment into reinforcement learning for playing games. In fact, Unity is developing some of the most cutting-edge technology in reinforcement learning and we will be working with this platform later. Unity does use C# for scripting but uses Python to build and train deep learning models.